Newly appointed professor: Data science can save food research decades
We can potentially save decades of food research by using data science to investigate where we have the greatest chance of finding the most significant results. It is a latent game changer when we want to convince society to eat a more plant-based diet, explains the newly appointed professor in computational food and health science at the University of Copenhagen.
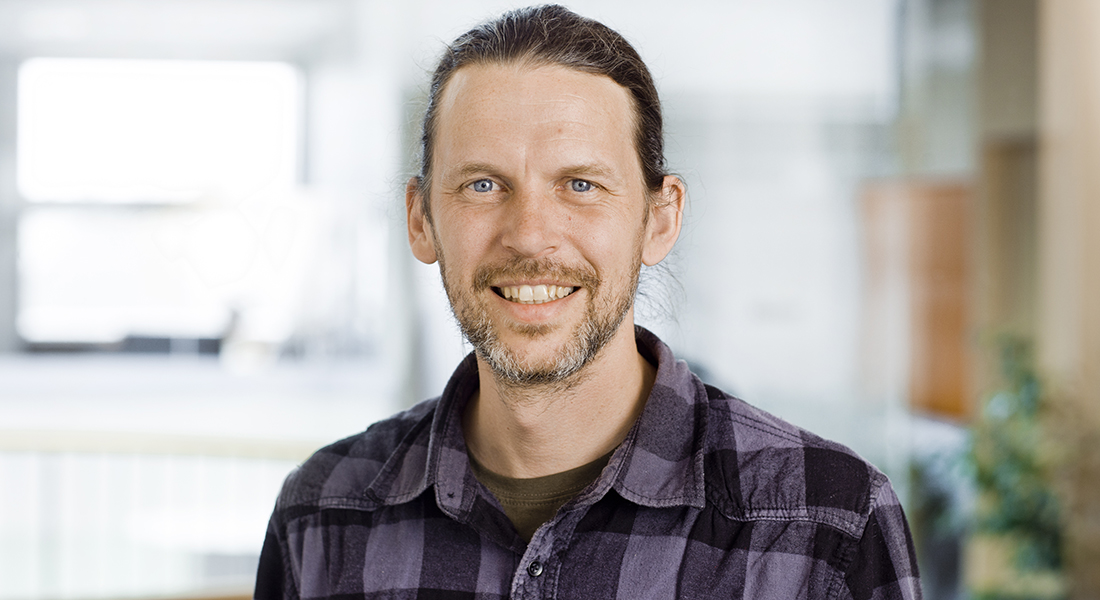
If food research can save years in finding the solutions that will create a more sustainable food system where we predominantly eat plant-based foods, it will be much needed. This requires an extensive research effort because plant proteins behave radically differently from animal proteins.
“It is equivalent to us going from building a house with materials that we have come to know over many years, to building the same house out of new materials that we could potentially react completely differently to. We now have to figure out how to fit the house together, create a good indoor climate, etc. If you look at it in a food historical context, this requires us to generate enormous amounts of new knowledge in an ultra-short time. Therefore, we must utilise the fantastic opportunities that data science gives us to find the optimal solutions must faster,” says Morten Arendt Rasmussen.
As of 1 January 2023, he has been appointed professor of computational food and health science, and divides his time between the Department of Food Science at the University of Copenhagen (UCPH FOOD) and the Danish Pediatric Asthma Center at Gentofte Hospital.
Why food research takes time
In food science, a research project is often developed in the following way: Some researchers get an idea for which they obtain funding. A research group is assembled, including PhD students who will help perform experiments in the laboratories and collect data along the way. Only when the laboratory work is almost finished do data scientists get involved, helping with the interpretation of the experiments/samples.
Researchers have traditionally worked in this way, by testing various hypotheses, which have been selected based on the literature and the researchers’ ideas, in order over a long period of time to arrive at the knowledge we have today.
Research projects
Morten Arendt Rasmussen conducts research using metabolomics and microbiomics, where the aim is to create a systemic characterisation of the biological system being studied – as opposed to studying changes in a single gene when it is affected by a specific substance.
He leads the research project FOODTOP, which strives to create a data model for personalised food pyramids for individuals suffering from inflammatory diseases such as asthma. Read more about FOODTOP.
In addition, he is co-leader of the TrACer project, which is a collaboration between COPSAC, UCPH FOOD and Simula Research Lab in Norway. He leads the five researchers working on the Danish part of the project. Read more about TrACer.
Read more about Morten Arendt Rasmussen on the University of Copenhagen’s staff database.
But with the new opportunities in data science, Morten Arendt Rasmussen sees great potential for speeding up the process in both consumer science and in food chemistry and microbiology, i.e., the actual processing of the food.
“We must use data both to find good ideas in the initial phase of the research projects, as well as now, in the final phase, where we use data to prove the hypotheses.”
Data-driven consumer science
“My plan is to develop computer models that can be used to extract knowledge about which foods consumers prefer from already existing databases on the web, with a view to speeding up green product development,” says Morten Arendt Rasmussen.
He teaches data analysis and statistics to e.g., students in the Master of Science (MSc) Food Innovation and Health Programme and sees a future where data analysis can be of great importance for consumer studies – not least when it comes to creating plant-based products that are both healthy and nutritious, and which consumers will eat because they are delicious.
“My idea is to use already existing consumer-oriented databases such as Allrecipes and Ratebeer as a starting point to determine which flavours and textures food-enthusiastic people around the world are experimenting with – and having success with,” he explains.
With the help of text-mining (where you extract information from huge amounts of text in order to decode contexts and patterns) and data-driven models, you will potentially be able to exploit this knowledge.
“Based on the results, we will be able to select the hypotheses that we spend time experimenting with in our laboratories more optimally. After all, there is a fantastically large resource of data created by people around the world and then assessed by others. We will be able to use it to get ideas and create new, plant-based products that are both healthy and safe, and which, based on the consumer-generated prior knowledge, will also have a good chance of winning the hearts of consumers, which is absolutely crucial for the success of the products,” says Morten Arendt Rasmussen.
Data-driven processing
Also, when it comes to processing, data science can potentially help food scientists make more informed choices before they go into the laboratory, believes Morten Arendt Rasmussen.
“Something we work with as food scientists is taking natural proteins and processing them into something else. This happens, for example, when we make cheese. Food processing almost always involves a modification of the proteins, and this has a major impact on texture and taste,” he explains.
The company DeepMind has created AlphaFold2 – a machine-learning model that, based on an amino acid sequence (amino acids are the chemical building blocks of all proteins; they sit like beads on a string, in what is called a sequence) can be used to predict how a “native” (i.e., natural) protein will fold into a three-dimensional structure. This has great significance for the protein’s biological activity. This formation, i.e., the three-dimensional structure of the protein in the raw material, is also the starting point for food processing, and can be used to predict how the protein will behave during e.g. heat treatment. So this can tell you about the properties of the end product.
“Proteins are one of the bottlenecks in our transition to a more plant-based diet, because plant proteins behave differently from animal proteins when we, for example, heat treat them, ferment them, cool them, whip them – everything we expose proteins to during the processing of the food,” says Morten Arendt Rasmussen. He continues:
“The hypothesis is that when AlphaFold2 can predict how a protein will fold, then this information is also relevant for how the protein behaves during processing. If we can predict this, we can screen all kinds of proteins in-silico (calculated via a computer) and thus uncover combinations of processing and raw materials in huge quantities that will be impossible to implement via wet-lab methods. We can then take the computer’s best solutions into the laboratory and test them. Such a research approach could probably save us decades,” says Morten Arendt Rasmussen.
Contact
Professor at the Department of Food Science at the University of Copenhagen (UCPH FOOD) Morten Arendt Rasmussen, mortenr@food.ku.dk
or
Communications officer at UCPH FOOD Lene Hundborg Koss, lene.h.koss@food.ku.dk